Smaller; Lighter; More Efficient
​
The Problem
​
Today, vehicle electrification is an important and accelerating market sector, but concerns persist around the cost and sustainability of electric powertrains.
How can motors be made smaller, lighter and more efficient?
​
Providing control inputs from realtime predictive models allows sensors and materials to be removed, reducing motor cost and weight - If the models are sufficiently accurate and efficient.

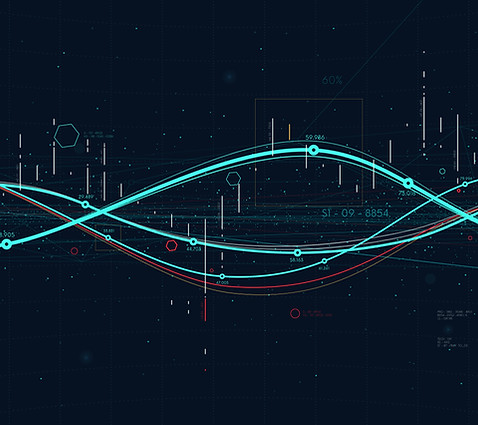
The Approach
We sourced around 140 hours of motor temperature and performance data made available by the University of Paderborn, and after exploring the relationships between variables, we built a series of predictive models for parameters of interest.
​
We developed solutions using a variety of modelling frameworks, from linear regression to neural networks, and compared models on predictive error and speed of computation to identify an optimum solution.
The Solution
​
Using this approach, we worked up a series of models for predicting temperatures in the rotor and stator (enabling finer motor control, and the removal of sensors).
​
The highest accuracy we achieved was around 96%, with sub 150 millisecond computation times on desktop PC hardware - Sufficiently performant for further development.
​
You can view the full project, including source code, models and reports, on our GitHub page.
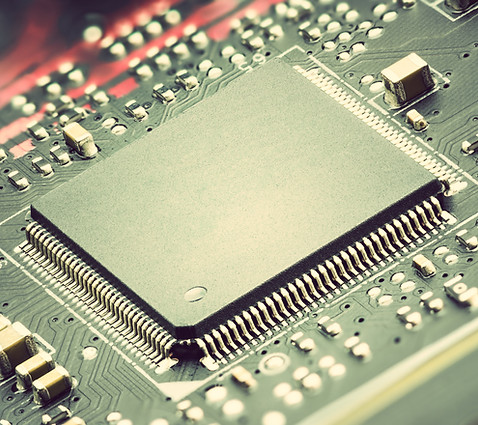